My first introduction to Large Language Model Artificial Intelligence came from my uncle, Mark Martinez, a state champion head football coach and exceptional high jump instructor. Being personally involved in academia, pursuing a doctorate, and coaching, I was fascinated by the potential of this new technology to enhance my daily work. That same evening, I challenged Chat GPT to produce an APA-formatted, 500-word essay on coaching and teaching methods, complete with up-to-date references. Upon initiating the request, I was immediately struck by the rapid generation of content, the AI’s general comprehension of my query, and the prospective boost to my efficiency this novel tool might offer.
Upon closer examination of the output, however, I noticed discrepancies in formatting, non-existent citations, and somewhat disjointed prose. While impressive, it was evident that the technology had limitations and still required human intervention.
Nevertheless, my interest was thoroughly piqued!
Since this initial introduction, I have spent considerable time immersed in the intersection of AI and education—giving presentations at conferences around the country, authoring articles, reading extensively on AI applications in higher education and K-12 settings, and even conducting a study with my colleagues involving over 300 educators on their AI usage and perceptions. Though I have been deep in the AI game for a few years now, I am blown away every day by a new feature or application for AI in my daily tasks.
The speed at which the technology has increased and improved has been dizzying, while the reception it has garnered from the education profession—including coaches—has been mixed at best. This article is meant to tamp down the concerns of those who think it will only dull the minds of the user and bring back to earth those who might think it is a band-aid that can be slapped on the wound of being undereducated.
The speed at which the technology has increased and improved has been dizzying, while the reception AI has garnered from the education profession—including coaches—has been mixed at best. Share on XIn my view, we do a disservice to our students, athletes, and clients if we choose to remain agnostic about AI usage. Even if you just have it double-check your work for errors, you are adding an extra level of assurance that you are offering the very best opportunities for growth to those who trust you enough to call you coach.
My Journey with AI in Coaching
The first time I used Claude Sonnet 3.7 to analyze my track team’s performance data, I felt like I had discovered the coach’s equivalent of a time machine. I uploaded several seasons worth of Excel sheets containing practice results, competition times, and individual progression metrics. Within minutes, Claude had processed years of training logs, competition results, and individual athlete progressions that would have taken me days to sift through manually. The insights were not just faster; they were also deeper, identifying patterns across seasons that I had completely missed despite my years of experience.
I was not replaced, as many said would happen. I was being enhanced.
Every time I start a task and think “this is taking too long,” I turn to AI for assistance. For example, when I collect data at practice, I usually write it down longhand on a notepad instead of entering it directly into Excel. This is significantly faster and helps the athletes get through their reps more efficiently. Previously, I would have to manually enter everything into Excel each evening. Now, I simply take a picture of my notepad and upload it to Claude, asking it to convert the data into an Excel file along with a brief analysis of the day’s results. After a couple of practice sessions, I can then ask the AI to analyze trends across multiple practices to determine if I need to adjust my training program going forward.
What I have learned is simple, but powerful: AI is not a gimmick. It is not a replacement for your content knowledge and coaching expertise, but it is not going anywhere.
When used effectively and efficiently, AI will not diminish our coaching abilities; instead, it will dramatically improve them. Share on XAs coaches, we need to embrace these tools for what they truly are, not what we fear they might be based on headlines or secondhand information. When used effectively and efficiently, AI will not diminish our coaching abilities; instead, it will dramatically improve them.
Understanding the AI Coaching Mindset
Before diving into specific applications, I want to lay out three fundamental aspects of working with AI as coaches.
1. How to Write Effective Prompts
The quality of the output from AI depends entirely on the input. Think of prompting as coaching AI—the more context, supporting documentation, and rationale you provide, the better your results.
I have found these strategies particularly effective.
- Provide rich context: Don’t just ask for a “sprint workout plan.” Instead, explain that you are working with high school juniors who are struggling with their start acceleration in the 100m, have been training for three years, and are currently in the late pre-competition phase.
- Include examples: If you want a certain format or style, provide an example. “I’m looking for a workout schedule similar to this one I used last season, but with modifications for athletes returning from minor hamstring issues…”
- Ask the AI for clarification: One of my favorite techniques is ending my initial prompt with: “Before we start, do you have any questions that will help clarify what I am asking you to do?”
Here is an example of a prompt I have used that follows these concepts.
“Last spring, I had the privilege of coaching our high school’s track and field sprint squad through what turned out to be a really interesting season. Throughout those months, I meticulously tracked our athletes’ progress—everything from their quick 10-meter fly times to their full 40-yard dashes. I have all that data saved, along with our complete training schedule that we followed throughout the season. Now, as I’m planning for next season, I find myself sitting here with these spreadsheets and training calendars, knowing there’s a story in this data that could help us do even better next year. The numbers tell me how each athlete progressed, but I’d love a fresh set of eyes to help me understand what patterns might be hiding in there.
What I want you to do is dive into this data with me—first to spot those patterns in how our athletes developed over the season, and then to look at how our training schedule might have influenced those outcomes. Maybe there were weeks where we hit the sweet spot with our training, or maybe there were missed opportunities we could capitalize on next time around? I’m about to share both the performance data and our training schedule from last season. Could you help me understand what story this data is telling, and then use those insights to suggest how we might refine our training approach for the upcoming season? I’m particularly interested in understanding not just what to change, but why those changes might help our athletes perform better.
All the data you need is attached as PDF documents.
Before we start, do you have any questions for me?”
And here is the output produced by Claude Sonnet 3.7:
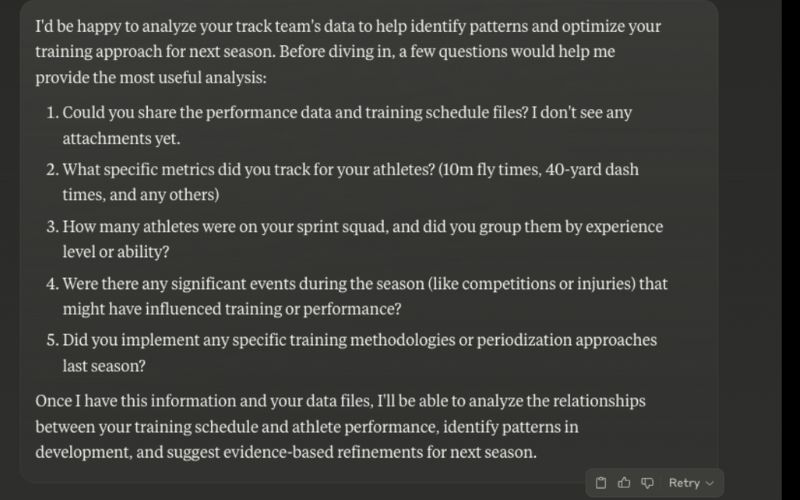
As you can see, it noticed I didn’t attach the files I said I would and asked for me to provide them, along with some more background information. So, in the next chat I answered Claude’s questions and provided the files for review.
Video 1. Screen recording of Claude 3.7 analyzing the inputs.
And here is what was produced:
“Based on my analysis of your track team’s data, I can see several interesting patterns in both your training schedule and athlete performance. Here’s what the data is telling us and how we might refine your approach for next season:
Key Training Schedule Insights
Your current training schedule follows a well-structured periodization approach with distinct phases:
1. Base Building Phase (Weeks 1-3)
- Gradual increase in Time Under Tension (TUT) from 11.5 to 14.5 seconds
- Focus on technique and introducing athletes to stimuli
2. Loading Phase (Weeks 4-5)
- Dramatic increase to peak volume (80 seconds TUT in week 5)
- The jump from week 3 to week 4 represents a 417% increase in TUT
3. Tapering Phase (Weeks 6-9)
- Steep initial decrease (44% drop from peak)
- Progressive reduction to 9 seconds TUT (93% below peak)
4. Reload Phase (Weeks 10-11)
- Brief increase in volume to maintain adaptations
- Still significantly below peak (43.5 seconds TUT)
5. Competition Phase (Weeks 12-13)
- Minimal TUT (5 and 3.5 seconds)
- Prioritizing freshness for championship competitions
Athlete Performance Analysis
Analyzing your athletes’ improvements across various metrics revealed:
1. Top Performers:
- Fastest male: ******
- Fastest female: ********
- Most improved male: ********* (13% average improvement)
- Most improved female: ******** (7.12% improvement)
2. Grade-Level Differences:
- Sophomores (grade 10) showed the highest average improvement (4.6%)
- Seniors (grade 12) showed the lowest average improvement (1.95%)
- Freshmen (grade 9) had the fastest average 40y dash times for males
3. Gender Differences:
- Male athletes showed greater variance in improvement rates
- Female athletes in grade 10 demonstrated particularly consistent improvements
Recommendations for Next Season
Based on these insights, here are specific refinements that could enhance your training approach:
- Base Building Phase
- Extend to 4 weeks to allow better adaptation, especially for younger athletes
- Implement more gradual TUT increases (e.g., 11.5→13.5→17→22) to prepare for the loading phase
- Consider differentiating volume between experienced and novice athletes
- Loading Phase
- Smooth the transition into peak loading (the 417% jump is likely too aggressive)
- Implement a more gradual build-up to peak (e.g., 22→40→60→80)
- Consider gender-specific loading profiles as your data shows different adaptation patterns
- Tapering Phase
- Create a more stepped taper rather than the sharp initial drop
- Consider a pattern like 80→65→50→35→20 seconds TUT
- Individualize taper rates for upperclassmen vs. underclassmen (data suggests different recovery needs)
- Targeted Development
- Customize training for different grade levels – your freshmen seem to respond well to speed work while sophomores show the best overall development
- The data suggests grade 10 athletes are in a “sweet spot” for improvement – consider how to capitalize on this developmental window
- For seniors, focus more on race-specific preparation and maintaining existing abilities
- Supplementary Changes
- Continue using the effective competition preparation phase approach (minimal TUT in final weeks)
- Consider shorter, more frequent lactate workouts during the loading phase instead of concentrated volume
- Add targeted acceleration development for athletes showing the largest gaps between fly times and push/dash times
Application for Next Season
To implement these changes effectively:
- Individualize training clusters – group athletes by development pattern rather than strictly by gender or event
- Monitor 10m fly times frequently as an early indicator of adaptation
- Introduce mini-tapers every 3-4 weeks to allow for better recovery
- Extend the base phase but maintain your excellent competition phase approach
- Create separate loading profiles for athletes based on grade level and recovery ability
These adjustments should help smooth out the dramatic transitions in your training schedule while still maintaining the successful periodization approach that seems to have worked well for your athletes overall.
Would you like me to elaborate on any particular aspect of these recommendations or discuss specific workout modifications for any phase of the season?”
As you can see, the output was substantial. But it was so because I allowed Claude to ask clarifying question to gain information that I might not have thought to include in the prompt.
2. How to Critique AI Outputs
Obtaining information from AI is not the finish line; it is just the starting block. As coaches, our expertise is crucial in evaluating what we receive.
- Verify the information: Did the AI “hallucinate” a research study or training technique that does not actually exist? AI can occasionally fabricate sources or combine information inaccurately.
In my work, AI most frequently hallucinates research that doesn’t exist. Because AI is programmed to be pleasing to the user, it will provide things it knows the user wants even when they don’t exist. That’s why I’ve incorporated specialized research AI tools like Consensus AI Scite, and PaperPal, which help me verify scientific claims and find legitimate research to ground my training methods.
In my work, AI most frequently hallucinates research that doesn't exist. Because AI is programmed to be pleasing to the user, it will provide things it knows the user wants even when they don't exist. Share on X- Compare with your expertise: Does the suggested approach align with your coaching philosophy and what you know to be effective for your specific athletes? AI provides suggestions based on patterns in its training data, but you know your athletes personally.
3. Understanding Your Coaching Domain
Arguably, having a deep understanding of the subject matter is the most crucial aspect of using AI well. Crafting effective prompts requires familiarity with the specific vocabulary related to the intended output of the prompt.
To effectively judge AI outputs, you must first have a comprehensive understanding of what quality looks like in your domain. You should be capable of creating what you are seeking without any AI assistance; AI simply helps you do it better or faster.
Think of AI as:
- An extra set of hands that can analyze piles of data in minutes instead of hours (I have experienced this firsthand, both when conducting qualitative thematic analysis as part of my doctoral work as well as crunching quantitative track practice data).
- An extra set of eyes that functions as one of the best editors you will ever work with. You can ask it to check for specific issues in your training plans or ensure that your approach aligns with the research or methodology you are basing it on.
Real-World Applications: AI in Track and Performance Coaching
Every season, I create a comprehensive practice plan and meticulously record data for each athlete on my team. I maintain a Google Sheet that houses all our athletes’ event records and practice metrics, such as 30m pushes from blocks, 10m flies, 10-yard flies, 40-yard dashes, and similar measurements. Each separate page of the Google Sheet represents a season and its collected data. Throughout the season, I track multiple data points to ensure that acceleration, max velocity, and speed endurance are improving as expected.
The data collected throughout the course of the season helped me adjust my plan, determine the needed rest days that I had not planned for, and make decisions about relay team composition. With AI, I’ve elevated this process significantly.
Now, I can feed years of historical team data into Claude or Grok—including past training plans and season outcomes—and ask it to identify trends, patterns, training gaps, or new training ideas tailored specifically to my team’s history. The beauty of this approach is that the suggestions are not based on generic articles about track teams in completely different contexts (like Florida!), they are individualized to my specific program and athletes!
This has always been the ultimate goal in coaching: to provide maximally-individualized attention in the most efficient way possible.
Furthermore, I have begun creating AI-assisted training plans for individual athletes. When I have a multi-event athlete plateauing while teammates continue to set PRs, I can ask the AI to help create a specialized plan (based on the team’s overarching framework) that addresses their specific needs.
Here’s a real-world example of how I might prompt AI to help with an athlete-specific issue:
“I have a sophomore male sprinter suffering from significant shin splints midway through our season. He’s an integral member of our 4x100m relay team that’s currently ranked top three in the state. I need to maintain his max velocity and speed endurance while reducing volume to help his shins heal. Based on my coaching philosophy of prioritizing quality over quantity and focusing on technical efficiency, what would you recommend to ensure he’s ready for the state meet in six weeks?”
Here’s what the AI provided in response:
“Based on your coaching philosophy of quality over quantity and technical efficiency, here’s a modified training approach for your sophomore sprinter with shin splints who needs to be ready for state:
SHORT-TERM RECOVERY PLAN (WEEK 1-2):
- Reduce ground contacts by 40-50% while maintaining intensity • Replace 2-3 track sessions with pool sprints or AlterG treadmill work if available
- • Limit starts to 3-5 quality reps twice weekly vs. normal volume
- • Focus on technical drills emphasizing proper dorsiflexion and foot strike
- Implement daily rehabilitation protocol: • Morning: 10-minute ice therapy and controlled ankle mobility work • Post-practice: 15-minute contrast bath therapy
- • Evening: Compression sleeves and elevation
- • 2-3x weekly sports massage targeting anterior compartment
- Cross-training to maintain fitness:
- • Upper body/core circuit training 4x weekly
- • Stationary bike intervals (30sec on/90sec off) for anaerobic maintenance •
- Aqua sprinting with resistance for power maintenance
TRANSITION PHASE (WEEK 3-4):
- Progressive return to track work: • Begin with straight-line acceleration work on softer surfaces (grass) • 60-70% normal volume with 100% intensity on technical days • Introduce curve running gradually with emphasis on relaxation • Continue relay exchange practice with walkthrough technique focus
- Modified speed endurance work: • Break longer repetitions into smaller segments with complete recovery • Example: Instead of 2x200m, use 2x(60m+60m+80m) with full recovery • This maintains quality while reducing continuous impact
STATE PREPARATION (WEEK 5-6):
- Race modeling with extended recovery: • Full-intensity relay leg simulations with 2x normal recovery time • 2-3 relay practice sessions weekly with emphasis on exchange efficiency • One time-trial simulation per week at 90-95% effort
- Peaking strategy: • Gradually return to normal volume by week 5, maintaining high intensity • Final 7 days: sharp reduction in volume while maintaining frequency • 48-hour pre-competition protocol emphasizing nervous system readiness
Throughout this plan, I would suggest daily monitoring of pain levels (1-10 scale), performing regular single-leg balance tests to assess neuromuscular control, and tracking 10m fly times once weekly to ensure velocity is maintained despite reduced volume.
This approach should allow your athlete to maintain his speed qualities while giving the shin splints adequate time to heal before the championship meet.”
What I appreciate about this response is how it addresses the immediate issue (shin splints) while keeping focus on the ultimate goal (state meet performance). The AI has broken down the six-week period into logical phases with specific, actionable recommendations that align with my coaching approach. I could implement this plan immediately, while still making adjustments based on how the athlete responds.
Or, even if I do not want AI to create a plan, I can use AI as a sounding board to help me get to the bottom of the issue.
Can I perform all these tasks without AI? Absolutely, I have for years. However, the improved quality of output when I provide sufficient context, combined with the time savings, makes it almost irresponsible not to use these tools. I have more time with my growing family and for my academic work and teaching, while my athletes benefit from more personalized training plans tailored to their individual needs.
AI as a Programming Partner
Not long ago, I had a conversation with one of my best friends, Reid Peters, who is the head strength coach at William and Mary College in Virginia. He told me about the mountain of data he collects on his athletes and how daunting it can be to sort through it all, compile it into actionable information, identify buckets that need to be filled, and then create 120 programs that meet the needs of his athletes—not just based on position groups, but based on the individual.
To address this common situation, let me provide an example of a prompt I would write to get the ball rolling in this monumental endeavor. I will then point out some subtleties.
“Hello! I am the head strength coach for a college football team preparing for our 12-week winter/spring offseason program beginning January 10th.
We have collected extensive performance data on our athletes over the fall season, and I need help analyzing patterns and creating individualized programming recommendations.
I will provide our testing data in CSV format with individual athlete results separated by position group (OL, DL, LB, DB, WR, QB, RB).
Our key performance indicators include: (Insert specific KPIs with measurement units – e.g., Trap Bar Deadlift (% bodyweight), 10yd Sprint (seconds), etc.)
I am attaching our departmental article outlining ideal KPI targets by position group, which explains how and why these specific metrics correlate with on-field performance.
Additional context:
- We have 3 strength coaches working with 85 scholarship athletes.
- Access to 12 power racks, 10 platforms, and a 40-yard indoor turf area.
- Training sessions limited to 75 minutes, 4 days/week.
- Previous year’s testing data included for comparison—Spring practice begins March 15th.
Based on this information, please:
- Identify the 3 most significant team-wide performance gaps compared to our ideal KPIs.
- For each position group, determine the most critical area needing improvement.
- For the athletes with the largest performance discrepancies, create individualized focus areas for my review.
- Recommend programming strategies that accommodate our facility/time constraints.
- Suggest a testing schedule to track progress throughout the 12-week period.
Before we start, do you have any questions about our testing protocols, athlete population, or what format would be most helpful for your analysis and recommendations?”
Notice that this prompt is exceptionally comprehensive. Its level of detail is such that an individual lacking substantial knowledge of the subject matter would struggle to generate it, as they would not possess the necessary understanding to formulate appropriate questions in the first place.
Now, let us break down why this is a good prompt:
- Clear context and timeline: The coach immediately establishes the specific program length (12 weeks) and provides important dates (January 10th start, March 15th, Spring practice). This temporal context is crucial for AI to understand the training windows.
- Data format specification: By mentioning the CSV format and position group organization, the coach prepares the AI for the expected data structure, which significantly improves the analysis accuracy.
- Specific metrics with units: The prompt specifies not only which KPIs to analyze but also includes their measurement units, eliminating ambiguity.
- Supporting documentation: The coach referenced an attached article explaining ideal targets and their relationship with performance, providing the AI with crucial background knowledge.
- Real-world constraints: The prompt outlines practical limitations (three coaches, 85 athletes, equipment availability, time constraints) that any recommendations must work within—something many coaches forget to specify.
- Historical context: By mentioning and providing the previous year’s data, the coach enables trend analysis rather than just a snapshot view.
- Clearly structured deliverables: The numbered list clearly defines exactly what the coach wants, in priority order.
- Open-ended follow-up invitation: The final question invites clarification, which often leads to important details being revealed before the analysis begins.
When writing a prompt, I want to be confident that it will produce actionable, contextualized recommendations rather than generic training advice. This example provides the AI with everything it needs to provide truly individualized insights that account for the complete coaching environment.
When writing a prompt, I want to be confident that it will produce actionable, contextualized recommendations rather than generic training advice. Share on XPro Tips for Coaches Using AI
Through trial, and lots of error, I’ve developed several strategies that significantly improve AI’s usefulness in coaching contexts. I put many of these tips into action in the example prompt above, but here they are broken down:
Upload Supporting Documents
Writing lengthy prompts is valuable, but often, your requests will be based on existing materials—articles, past training plans, or images. Instead of re-typing everything, simply upload those documents along with a brief prompt.
For example, I teach a speed coaching course at my university and offer it as a standalone certification as well. For these courses, I have written two e-books defining my entire approach to speed development and the factors I consider when creating training plans. Rather than rewriting my philosophy each time I need to adjust something, I simply upload my e-books into the AI chat as a starting point. The AI then has everything it needs to know about my coaching approach to help me make programmatic decisions that are aligned with my methodology.
You do not need to write books for this to work, but having solid, long-form prompts that you know you will use again tucked away is a good idea. This leads to my next point.
Save Effective Prompts
I maintain a dedicated folder on my computer for prompts that generate excellent results. From revamping course syllabi to tracking my speed clients’ progress, I preserve the prompts that consistently deliver value to my end user.
This saves a tremendous amount of time when addressing similar coaching challenges in the future. I will include a bunch of useful prompts at the end of this article.
Choose the Right AI Tools
While many options exist, I recommend focusing on established platforms.
- Grok offers impressive capabilities, particularly for data analysis and real time information.
- Claude is the tool I’m most familiar with and find exceptionally helpful for coaching applications.
- ChatGPT remains solid, although it has also received the most attention as the first widely-adopted platform.
Stay informed about updates to each platform to determine which one best suits your specific coaching needs. Consider factors like:
- Internet connectivity for accessing real-time information.
- Document upload capabilities.
- Data visualization features.
- Mobile accessibility for sideline use.
Differentiating for Individual Athletes Beyond Track
Although my background is in track, AI’s potential extends across all coaching domains. Here are some applications beyond the track that leverage the same individualization principles.
For Recovery Protocols
Recovery is increasingly recognized as crucial to performance, yet it is highly individualized. AI can help process multiple data points, sleep quality, subjective fatigue ratings, HRV measurements, and previous recovery responses to suggest personalized recovery protocols.
A wrestler cutting weight while maintaining performance could receive AI-generated nutrition and recovery plans that account for their specific metabolic needs, training load, and competition schedule, all based on their individual data, rather than generic recommendations.
For Mental Performance
Mental skills coaches can use AI to identify patterns in performance anxiety or concentration issues across different competition settings. By analyzing competition journals, performance metrics, and pre-competition routines, AI can help identify triggers and suggest personalized mental skills interventions for athletes.
A golfer struggling with performance consistency could receive an AI-assisted analysis identifying that their putting performance decreases specifically in the final three holes when leading, which might take months to identify through traditional coaching methods alone.
Getting Started with AI in Your Coaching Practice
If you’ve never used AI tools before, the prospect might seem daunting. Here’s a simple roadmap to get you started without feeling overwhelmed:
Step 1: Choose Your First AI Tool
Start with one platform rather than trying several simultaneously. I recommend Claude or ChatGPT as beginner-friendly options. Both have free tiers that allow you to experiment before committing financially.
- Sign up at claude.ai or chat.openai.com.
- Take 10 minutes to explore the interface.
- Look for options to upload documents (this will be valuable later).
Step 2: Begin with a Simple, Low-Stakes Task
Your first AI interaction doesn’t need to be revolutionary. Here are three simple starter tasks:
- Ask it to explain a coaching concept you already understand well. This helps you gauge the AI’s baseline knowledge in your domain.
- Have it proofread an existing document like a practice plan or parent communication. Type: “Please proofread this document for clarity, grammar, and tone, suggesting improvements where appropriate.”
- Draft a standard email template you send frequently. For example: “Create an email template I can send to athletes who miss practice, emphasizing accountability while maintaining a supportive tone.”
Step 3: Try a Coaching-Specific Prompt
Once comfortable with the basics, try this simple prompt template:
“I am a (sport) coach working with (age/level) athletes. I need help creating a (practice plan/drill/feedback script) focused on (specific skill or concept). My athletes are currently struggling with (specific issue). Their strengths include (strengths). Our practice environment includes (equipment/space/time constraints). Can you provide a detailed (practice plan/drill/feedback script) that addresses these needs?”
Fill in the parentheses with your specific information, and you’ll receive a customized response you can evaluate and adapt.
Step 4: Incorporate Document Uploads
Once you’re comfortable with basic prompts, try uploading a relevant document—perhaps a research article about your sport or a previous practice plan. Then ask:
“Based on this document and my context as a (sport) coach with (level) athletes, what are 3 key ideas I could implement in my next practice?”
Step 5: Create a Prompt Journal
Start a simple document or note on your phone where you save prompts that worked well. Include:
- The exact prompt text.
- What you liked about the response.
- How you might modify it next time.
Here are 12 specific prompts that track coaches focused on short sprints might find particularly valuable:
- “Analyze these 10m, 30m, and 60m sprint times from our last three practices and identify which athletes are showing the greatest improvements in acceleration vs. top-end speed.”
- “Based on these results from our 4x100m relay team and their splits, calculate the exchange efficiency for each handoff and suggest technical adjustments.”
- “Create a periodized 8-week plan for improving 200m performance for a sprinter who’s strong in acceleration but struggles with maintaining speed on the curve. The program I have been using doesn’t seem to meet the needs of this athlete. They are also a soccer player who practices MWF with their soccer team for 2 hours, and then on TTH, they practice with us”
- “Compare these block start reaction times and 10m split times from practice to identify correlations between stance setup and initial acceleration. These are from the same athlete”
- “Come up with three cues to improve arm mechanics for a 100m sprinter who drops their elbows during the final 30m of their race. My go-to cues don’t seem to resonate”
- “Analyze this season’s workout data for my top female 100m sprinter and identify the optimal volume-intensity balance that preceded her best performances.”
- “Create a visualization template for tracking weekly training loads across acceleration, max velocity, and speed endurance sessions throughout a 16-week season and apply it to the schedule I have provided.”
- “Evaluate this video analysis data of ground contact times and stride frequency across my sprint group and recommend individualized cues for each athlete.”
- “Generate a decision tree for modifying sprint workouts based on wind conditions ranging from +3.0 to -3.0 m/s.”
- “Design a spreadsheet formula that automatically calculates speed decay percentage between first and second 100m in a 200m race.”
- “Convert these handwritten practice notes into an organized Excel format with conditional formatting that highlights values outside the expected range.”
- “Create an athlete-friendly explanation of how we’ll use GPS data from practice to individualize their flying sprint distances based on their stride patterns.”
- “Here are the results from our last meet, create an email I can send out to parents that highlight PRs, wins, and other accomplishments.”
Saving these prompts can become a valuable asset as you build your AI coaching arsenal, eliminating the need to start from scratch each time you sit down at your computer.
Remember, your first attempts don’t need to be perfect. The goal is to start small, experiment regularly, and gradually integrate AI into aspects of your coaching where it adds genuine value. Like any new coaching tool, proficiency comes with consistent practice and reflection.
The Future of AI in Coaching
As AI tools continue to evolve, we are likely to see even more specialized applications for coaches. Things like real-time analysis of practice footage with immediate feedback suggestions, predictive injury prevention based on subtle changes in movement patterns, comprehensive development tracking that integrates physical, technical, tactical, and psychological factors, and virtual assistant capabilities that handle administrative tasks while coaches focus on athlete relationships.
The coaches who thrive will be those who leverage AI to handle time-consuming tasks while they focus on what matters most: the human connection, motivation, and nuanced guidance that no algorithm can provide. Share on XHowever, the fundamental principle remains: AI will never replace quality coaching itself. The coaches who thrive will be those who leverage AI to handle the time-consuming analytical tasks while they focus on what matters most: the human connection, motivation, and nuanced guidance that no algorithm, prompt, or engine can provide.
Final Thoughts: Embracing the AI Coaching Revolution
Let me be completely transparent: adopting AI in your coaching practice requires an initial investment of time and effort. Learning to write effective prompts, critically evaluating outputs, and building systems that work for specific contexts does not happen overnight. Just as you have continuously evolved your coaching methods throughout your career, incorporating AI represents the next evolution, not a replacement of your hard-earned wisdom but an amplification of it!
Coaches who resist this change will eventually find themselves at a competitive disadvantage. I just had a student ask me last week why more professors do not teach them how to use AI effectively. She said, “They know we will need to know how to use it, but they refuse to learn how to use it themselves or teach us how to use it! It’s putting us at a disadvantage.” Do not put your athletes at a disadvantage by not taking advantage of the tools at your disposal. Coaches who embrace it thoughtfully will discover what I have: more time for what matters most, deeper insights into their athletes’ needs, and ultimately, better performance outcomes.
I encourage you to start small, experiment consistently, and approach AI with the same growth mindset you foster in your athletes. The future of coaching is not human or artificial intelligence; it is the powerful combination of both!
P.S
If you would like to further this conversation, I would love to be of assistance! You can reach me at [email protected]